This is a poster paper we have presented at the 37th Medicinal Chemistry Symposium held in Hachioji, Tokyo Japan from the 27th to 29th of November 2019. The PDF of the poster can be accessed through the link at the end of the blog.
The paper is about the use of Chemical Genomics-Based Virtual Screening (CGBVS) to find compounds in the ChEMBL25 database having potential inhibitory activity against protein-protein interaction.
What is CGBVS anyway?
CGBVS is a machine learning-based method for predicting the activity of a compound based on the binding pattern obtained from the interaction information (chemical genomics information) between the protein (biological space) and the compound (chemical space).
There are huge amounts of chemical genomics information that can be obtained from public databases, such as ChEMBL and Uniprot, that can be used as training data to create predictive models. The concept of the technique is summarized in the following figure.
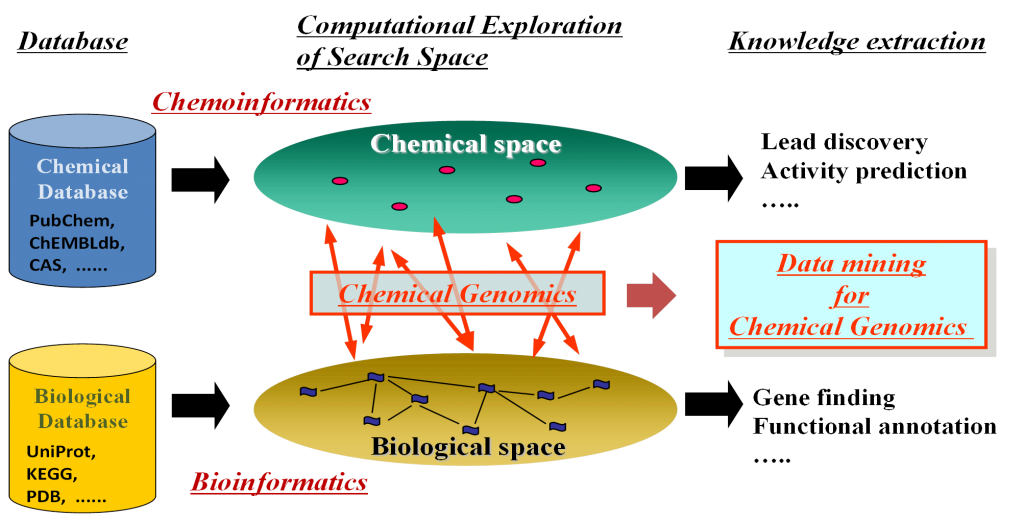
CGBVS was developed in the lab of Professor Yasushi Okuno during his tenure at the Graduate School of Pharmaceutical Sciences at Kyoto University. He has since moved to the Graduate School of Medicine at the same University. His expertise was mainly in the field of bio- and chemoinformatics in which he has produced many publications.
The steps leading to the creation of predictive models are illustrated in the figure below. It is worth noting that the technique utilized support vector machines (SVM) which is still considered to be one of the reliable approaches in the field of chemoinformatics.
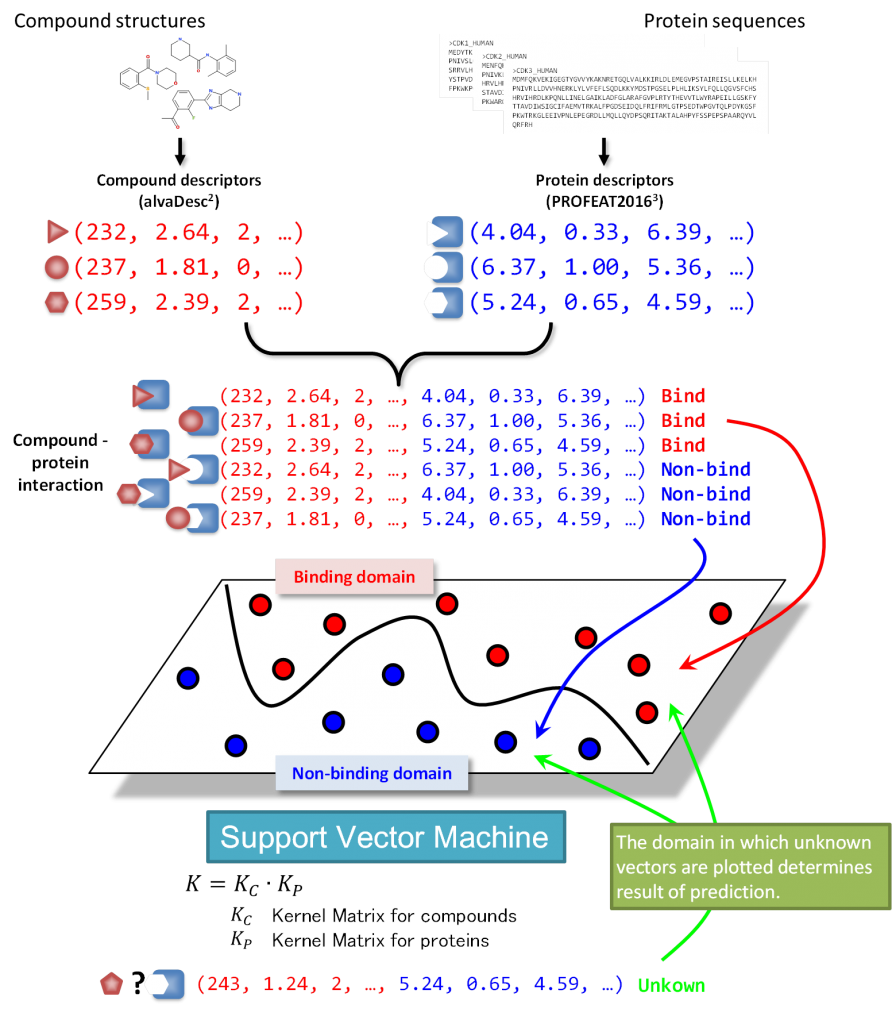
There are currently 8 available predictive models that are used to screen against protein targets corresponding to GPCRs, kinases, ion channels, nuclear receptors, proteases, transporters, cytochrome P450 and PPI related proteins. Cytochrome P450 and PPI models were only recently added to the list, that is, after this poster paper was presented in the recent Medicinal Chemistry Symposium.
These models are created requiring only 2d compound descriptors, protein descriptors, and compound-protein interaction data. Compound descriptors are generated using the application alvaDesc, which is the successor to the widely used DRAGON application. On the other hand, the protein descriptors are generated using the PROFEAT 2016 web server. The compound protein interaction data were mainly obtained from the ChEMBL25 database but for the purpose of this current research, the PPI predictive model we have created was based on the TIMBAL database which is a database mainly catering to PPI data.
The TIMBAL database is basically a mixture of different types of assay data pertaining to protein-protein interaction in which activity values are presented as IC50, Ki, Kd, or %Inh. Activity units were presented as % or molarity units (mM, uM, nM, pM). We have only selected data whose activity values are presented in molarity units and converted all values to uM. For the creation of training data sets, we have set positive and negative cutoffs at <=10 uM and >=15 uM, respectively. All in all, the total number of interaction data was 8,602 for positive and 1,680 for negative.
The following figure shows the flow for the creation of the PPI model.
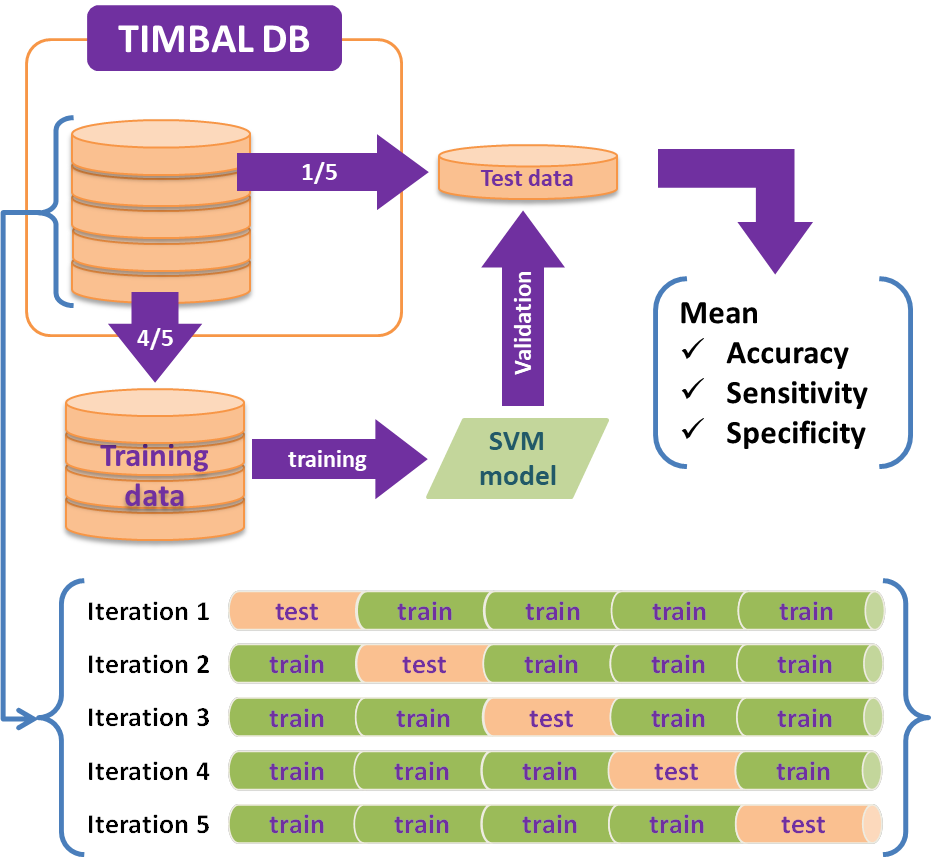
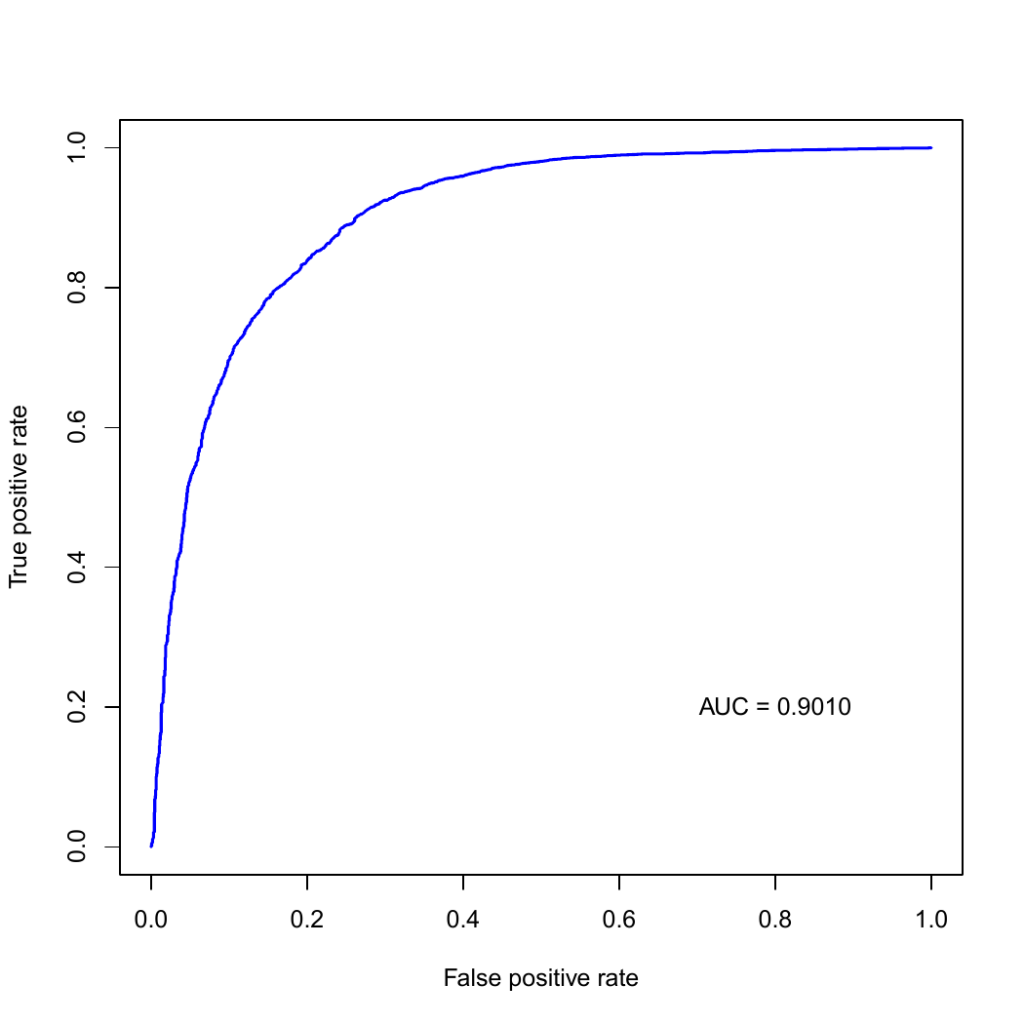
Based on the target protein list we extracted from the TIMBAL database, we obtained compounds from the ChEMBL25 database that have shown activities against the targets in the abovementioned protein list. We selected only the compounds that are present only in the ChEMBL25 database, that is, we removed the compounds that are found to be existing in the training data used to create the PPI model. In total, we have obtained 67,422 compounds which we screened against the PPI model.
CGBVS scores are, generally, values between 0 and 1, and values greater than or equal to 0.5 are considered to be positive or, simply put, they have potential activity against the corresponding target protein. The table below lists the number of positive compounds for each target protein. Although there were 71 target proteins registered in the PPI model, only 46 of them were present in the training data, hence, CGBVS calculation included only those 46 proteins.
Target Protein | No. of Compounds with activity |
---|---|
ITB3_HUMAN | 7655 |
BCL_HUMAN | 7106 |
BRD4_HUMAN | 5043 |
B2CLI_HUMAN | 4986 |
MDM2_HUMAN | 4848 |
ITB1_HUMAN | 4824 |
ITA4_HUMAN | 4009 |
ITA2B_HUMAN | 3281 |
ITB2_HUMAN | 3141 |
BAD_HUMAN | 2769 |
ITB7_HUMAN | 2126 |
TTHY_HUMAN | 2084 |
PPIA_HUMAN | 1956 |
HIF1A_HUMAN | 1884 |
ITB5_HUMAN | 1491 |
FKB1A_HUMAN | 1262 |
XIAP_HUMAN | 1166 |
CTNBI_HUMAN | 1159 |
BRD2_HUMAN | 715 |
ITA5_HUMAN | 569 |
ITB6_HUMAN | 537 |
TNFA_HUMAN | 195 |
MEN1_HUMAN | 122 |
TAB1_HUMAN | 118 |
IL2_HUMAN | 82 |
PPIB_HUMAN | 81 |
STAT3_HUMAN | 72 |
RAD51_HUMAN | 71 |
VEGFA_HUMAN | 57 |
S10AA_HUMAN | 51 |
ANXA2_HUMAN | 476 |
BRDT_HUMAN | 32 |
NRP1_HUMAN | 32 |
MDM4_HUMAN | 28 |
KEAP1_HUMAN | 20 |
TF7L2_HUMAN | 20 |
S100B_HUMAN | 18 |
MED23_HUMAN | 5 |
ELF3_HUMAN | 4 |
ITA2_HUMAN | 3 |
TNR5_HUMAN | 3 |
XPO1_HUMAN | 3 |
RAC1_HUMAN | 2 |
RASK_HUMAN | 2 |
CLH1_HUMAN | 0 |
MYC_HUMAN | 0 |
Among the compounds having positive results, we presented 4 of them showing activity against Bcl-2 (BCL2_HUMAN) and Bcl-xl (BAD_HUMAN) proteins. IC50 values are obtained from the ChEMBL25 database. I would like to emphasize that Venetoclax has been in the drug market for a while and is known to be an active Bcl-2 inhibitor. It is generally used to treat adult patients with chronic lymphocytic leukemia (CLL).
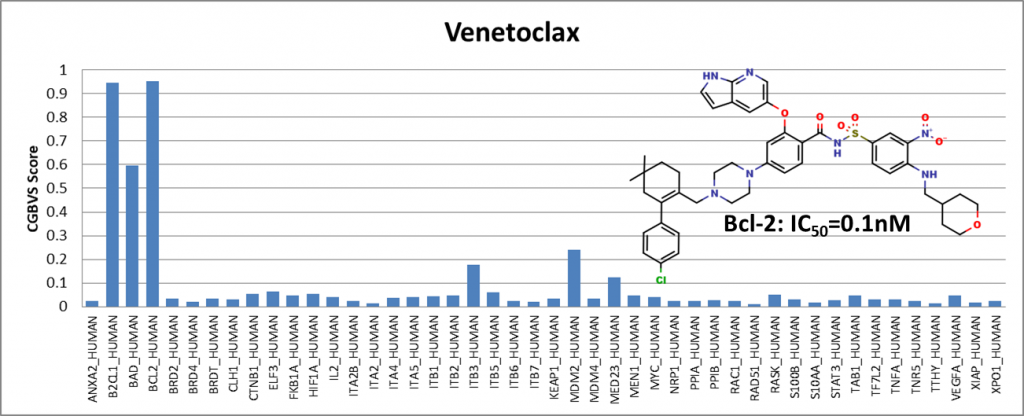
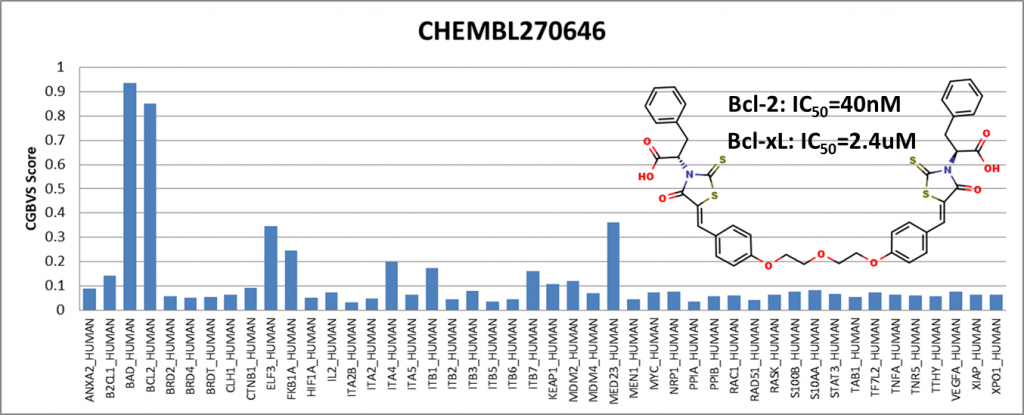
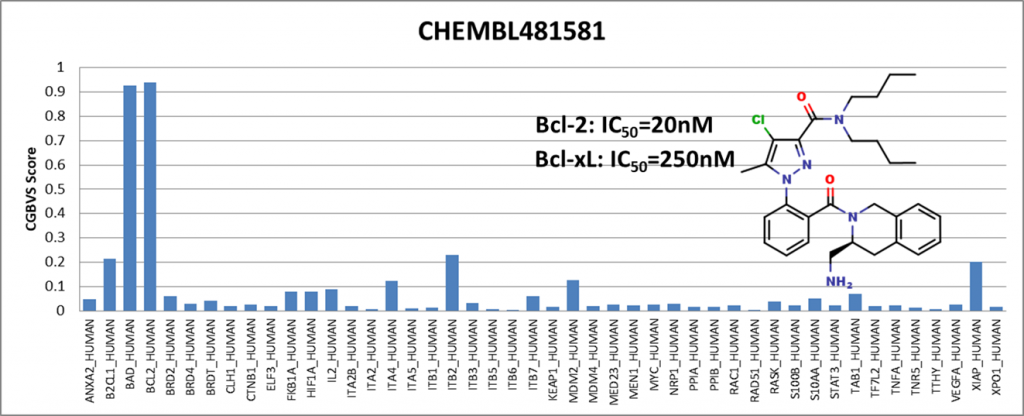
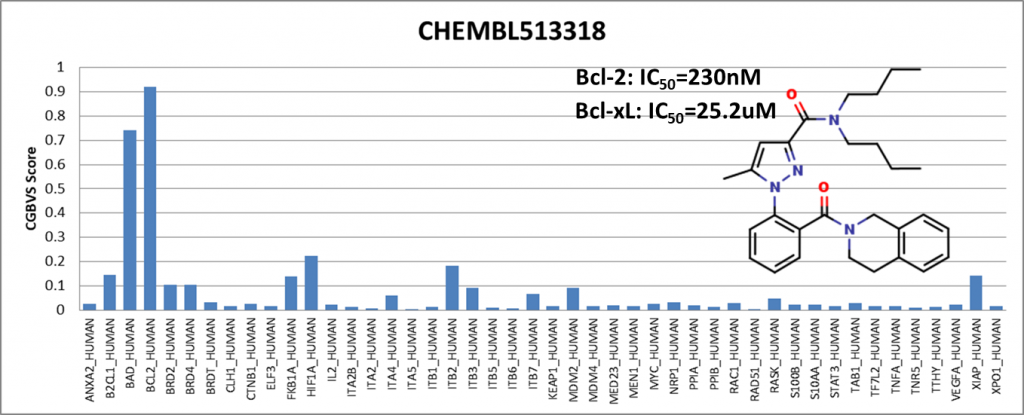
We have shown that even though the training data did not include compounds from the ChEMBL25 database, the PPI model showed sensitivity with respect to Bcl-2 protein.
Category: CGBVS/CzeekS, DRAGON/alvaDesc, Machine Learning